บริการเอพีไอเพื่อการจำแนกระดับความเสี่ยงการเกิดแผลกดทับของผู้ป่วยติดเตียง โดยบูรณาการการเรียนรู้ของเครื่องและชุดเซนเซอร์ไร้สาย
คำสำคัญ:
บริการเอพีไอ, แผลกดทับ, ผู้ป่วยติดเตียง, การเรียนรู้ของเครื่อง, เซนเซอร์ MPU-650บทคัดย่อ
แผลกดทับเป็นสาเหตุสำคัญที่ส่งผลให้ผู้ป่วยติดเชื้อในกระแสเลือด จนทำให้ผู้ป่วยเสียชีวิตในเวลาอันรวดเร็ว จึงเป็นโจทย์สำคัญในงานวิจัยนี้ .โดยมีวัตถุประสงค์เพื่อค้นหาคุณลักษณะของชุดข้อมูลในการจำแนกระดับความเสี่ยงการเกิดแผลกดทับของผู้ป่วยติดเตียง เพื่อพัฒนาและประเมินประสิทธิภาพของโมเดลและบริการเอพีไอเพื่อการจำแนกระดับความเสี่ยงการเกิดแผลกดทับของผู้ป่วยติดเตียง โดยการเรียนรู้ของเครื่องและเซนเซอร์ รวบรวมชุดข้อมูลด้วยแบบประเมินของบาร์เดน ร่วมกับใช้เซนเซอร์เพื่อตรวจจับการเคลื่อนไหวด้วย MPU-650 กับผู้ป่วยติดเตียงในกลุ่มโรคไม่ติดต่อเรื้อรัง โรงพยาบาลวัดโบสถ์ จังหวัดพิษณุโลก จำนวน 78 คน ทดสอบประสิทธิภาพการจำแนกระดับความเสี่ยงการเกิดแผลกดทับเพื่อเลือกอัลกอริธึมที่มีประสิทธิภาพมากที่สุด ได้แก่ อัลกอริธึม KNN, WKNN การเพิ่มเทคนิคการเลือกคุณลักษณะ และเซนเซอร์ ผลการวิจัยพบว่า การเพิ่มคุณลักษณะจากเซนเซอร์ในการนับจำนวนการขยับร่างกาย/เปลี่ยนท่าทาง ในโมเดล KNN กับ Information gain แบบ 5 คุณลักษณะช่วยเพิ่มความถูกต้องในการทำนาย เฉลี่ยร้อยละ 7 โดยให้ความถูกต้องสูงสุด 93.6% ที่ k = 3 และ 7 สามารถนำมาพัฒนาบริการเอพีไอต่อเชื่อมกับโมเดลและเซนเซอร์ เพื่อรองรับการเข้าถึงจากซอฟต์แวร์ภายนอกที่พัฒนาด้วยภาษา PHP
References
Akyildiz, I. F., Su, W., Sankarasubramaniam, Y., & Cayirci, E. (2002). Wireless sensor networks: A survey. Computer Networks, 38(4), 393-422.
Arpita, J., & Ashish, M. (2018). Analysis of K-Nearest Neighbor technique for breast cancer disease classification. International Journal of Recent Scientific Research, 9(4I), 26126-26130.
Bergstrom, N., Braden, B. J., Laguzza, A., & Holman, V. (1987). The Braden Scale for Predicting Pressure Sore Risk. Nursing Research, 36(4), 205-210.
Bo, S., Junping, D., & Tian, G. (2009). Study on the improvement of K-Nearest-Neighbor algorithm. In Proceedings of the International Conference on Artificial Intelligence and Computational Intelligence (AICI 09) (Vol. 4, pp. 390-393). IEEE Computer Society. https://doi.org/10.1109/AICI.2009.312
Fielding, R. T. (2000). Architectural styles and the design of network-based software architectures (Doctoral dissertation, University of California, Irvine).
Imandoust, S. B., & Bolandraftar, M. (2013). Application of K-Nearest Neighbor (KNN) approach for predicting economic events: Theoretical background. International Journal of Engineering Research and Applications, 3(5), 605-610.
Jabbar, M. A., Deekshatulu, B. L., & Chandra, P. (2013). Classification of heart disease using K-Nearest Neighbor and Genetic Algorithm. In International Conference on Computational Intelligence: Modeling Techniques and Applications (CIMTA) (pp. 85-94).
Jiricka, M. K., Ryan, P., Carvalho, M. A., & Bukvich, J. (1995). Pressure ulcer risk factors in an ICU population. American Journal of Critical Care, 4(5), 361-367.
Mitchell, T. M. (1997). Machine learning. McGraw-Hill.
Modi, A., Shah, K., Shah, S., Patel, S., & Shah, M. (2024). Sentiment analysis of Twitter feeds using flask environment: A superior application of data analysis. Annals of Data Science, 11(1), 159-180.
Watbot Hospital. (2016). Interview contents for gathering problems in health care system of patients lying on bed. Phitsanulok: Watbot Hospital.
Zeynu, S., & Patil, S. (2018). Prediction of chronic kidney disease using data mining feature selection and ensemble method. International Journal of Pure and Applied Mathematics, 118(24).
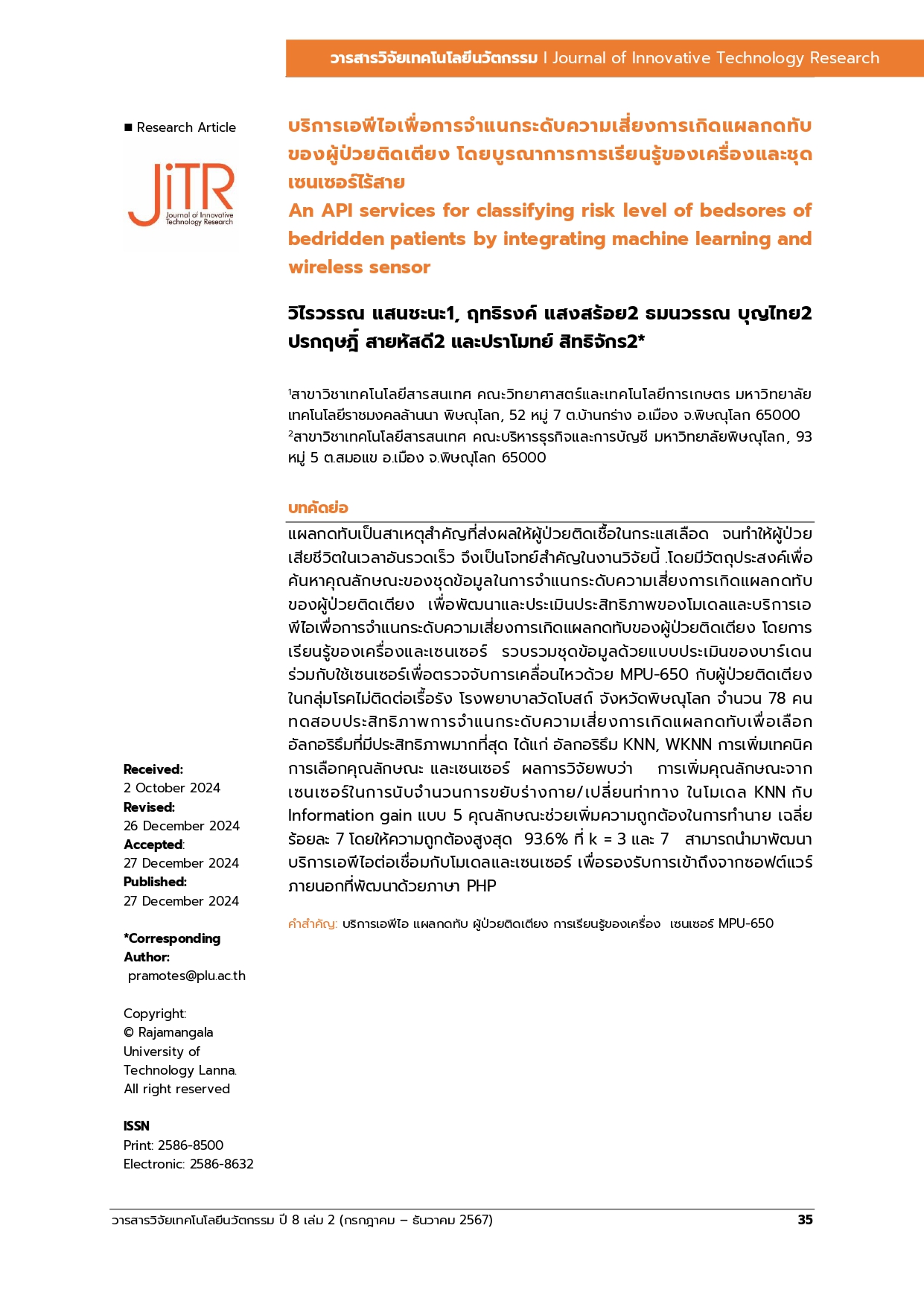
Downloads
เผยแพร่แล้ว
How to Cite
ฉบับ
บท
License
Copyright (c) 2025 สถาบันวิจัยและพัฒนา มหาวิทยาลัยเทคโนโลยีราชมงคลล้านนา

This work is licensed under a Creative Commons Attribution-NonCommercial-NoDerivatives 4.0 International License.
กองบรรณาธิการขอสงวนสิทธิ์ในการปรับปรุงแก้ไขตัวอักษรและค่าสะกดต่าง ๆ ที่ไม่ถูกต้อง และต้นฉบับที่ได้รับการตีพิมพ์ในวารสารเทคโนโลยีและนวัตกรรม ถือเป็นกรรมสิทธิ์ของสถาบันวิจัยและพัฒนา มหาวิทยาลัยเทคโนโลยีราชมงคลล้านนา และผลการพิจารณาคัดเลือกบทความตีพิมพ์ในวารสารให้ถือเป็นมติของกองบรรณาธิการเป็นที่สิ้นสุด